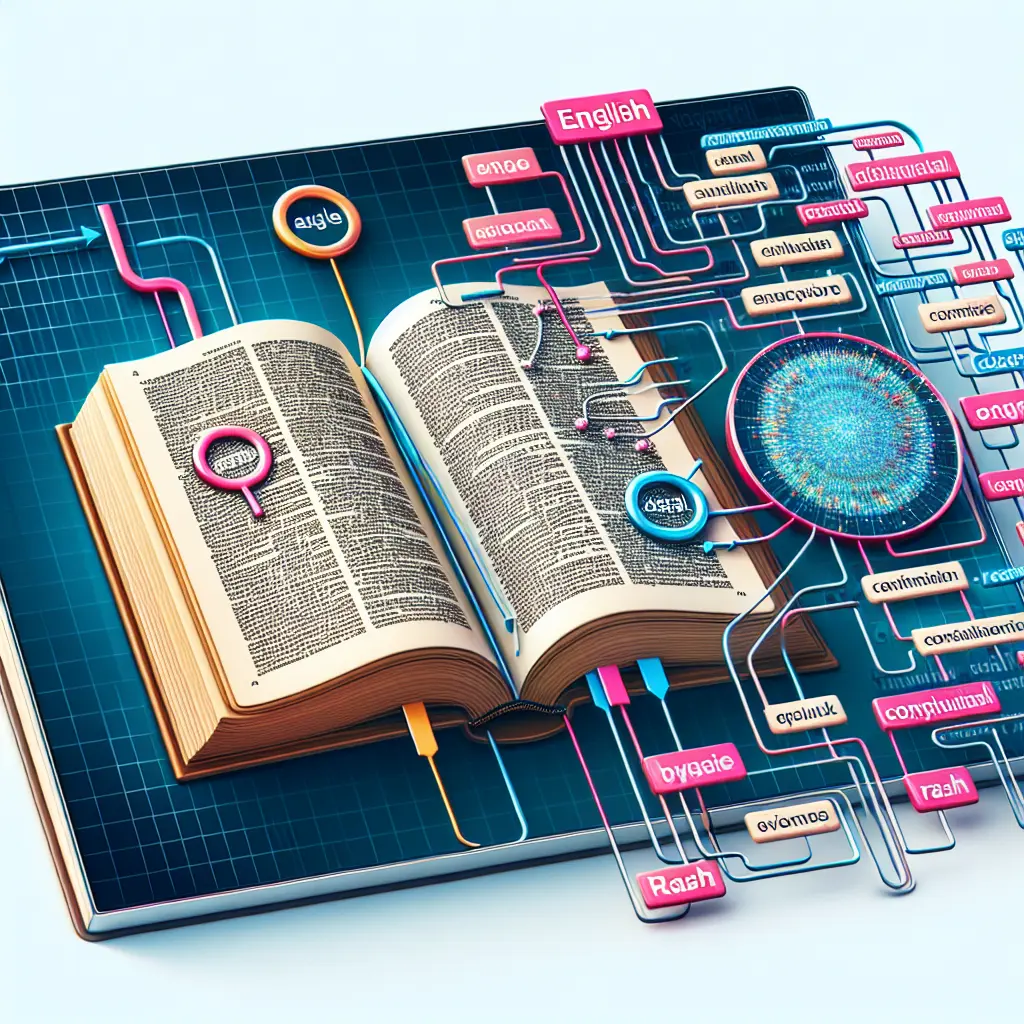
Exploring Innovative Approaches in English Discourse Analysis: Rasch and CRF Models
In the ever-evolving field of language learning and linguistics, new methodologies and technologies continuously shape our understanding and teaching of languages. A recent study highlights the combination of the Rasch measurement model with Conditional Random Field (CRF) models to provide a more nuanced analysis of English discourse.
Understanding the Rasch Measurement Model
The Rasch measurement model is a well-established tool in linguistics, primarily used for evaluating qualitative data in a structured, quantitative manner. It transforms qualitative assessments into measurable metrics, allowing educators and linguists to gain deeper insights into language proficiency and comprehension.
- Scalability: By converting qualitative data into scalable metrics, the Rasch model facilitates the comparison of different discourse analyses.
- Precision: It offers precise feedback, making it invaluable for educational settings where understanding specific strengths and weaknesses in language skills is crucial.
The Role of Conditional Random Field (CRF) Models
CRF models enhance discourse analysis by providing a robust framework for predicting sequences. In linguistics, these models are particularly effective in:
- Sequence Labeling: This is vital for understanding sentence structures, identifying parts of speech, and analyzing syntactic patterns.
- Contextual Analysis: CRFs excel at incorporating context into analysis, which is essential when deciphering the subtleties of language use and meaning.
Synergizing Rasch and CRF Models
By integrating the Rasch measurement model with CRF models, researchers can achieve a comprehensive approach to discourse analysis that combines both quantitative precision and contextual depth. The synergy between these models can lead to:
Enhanced Feedback: Educators can offer more detailed feedback to learners, addressing both statistical performance and contextual understanding.
Improved Learning Outcomes: By understanding linguistic patterns better, educators can tailor their teaching strategies to meet specific learning needs.
Advanced Research Applications: This combination opens new avenues for linguistic research, allowing for more sophisticated analyses of language usage across different contexts and cultures.
Conclusion
The intersection of the Rasch measurement model and CRF models marks a significant advancement in the field of language learning and linguistics. By leveraging these tools, educators and researchers can provide richer insights into language proficiency, ultimately fostering more effective teaching methods and improved learning experiences.
As we continue to explore these innovative approaches, it's crucial to embrace such advancements to keep pace with the dynamic nature of language and its myriad forms of expression.
For a deeper dive into this fascinating study, you can access the full article here.
Until next time, may your journey through the world of words be as enlightening as it is enriching.
Author: Calvin Broadmoor